I'll be instructing a 4-week online course Forecasting Analytics at Statistics.com. Learn about popular forecasting methods and how to implement them in practice through a hands-on online course. This is a practical course that introduces forecasting methods, performance evaluation, and much more. The course is of interest to practitioners and researchers in business, environmental sciences, agriculture, tourism and any field that collects time series data. It is also useful for instructors developing a new forecasting analytics course. The text for the course is Practical Time Series Forecasting: A Hands-On Guide, available globally through Amazon and as a Kindle book.
News
Online course Forecasting Analytics opening March 29.

2-Day Crash Course: Effective Data Presentation (Thimphu, 28-29 March)

I'll be instructing a 2-day crash course on Effective Data Presentation on March 28-29, 2013 at the Rigsum Institute in Thimphu. This course is a must for those who collect, analyze or present data or statistics. We will use data from Bhutan and software that is accessible to many (Microsoft Excel and Tableau Public). Follow the link for more details and registration.
"To Explain or To Predict?" - seminar at University of Peradeniya, Sri Lanka (Feb 23, 2013)
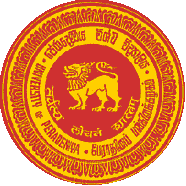
I'll be giving a guest lecture on "To Explain or To Predict?" at the Postgraduate Institute of Science (PGIS) at the University of Peradeniya in Kandy, Sri Lanka. See the abstract and further details.
Too Big To Fail: Large Samples and the P-Value Problem -- forthcoming in ISR
This weekend an important paper that I co-author with Hank Lucas and Mingfeng Lin has been accepted to the prestigious journal Information Systems Research. The paper, entitled "Too Big to Fail: Large Samples and the P-Value Problem" describes a critical challenge that occurs in modeling large samples. Publications in fields such as Information Systems as well as other social sciences have begun to rely on very large samples for testing theories. The problem is that using "good old statistical significance" for deriving practical conclusions can lead researchers astray because with a large sample even very small effects will be statistically significant at traditional levels.
I hope that the publication of the paper creates more awareness and opens a discussion about new ways for carrying out empirical research in the social sciences in the advent of "big data".
Here's the abstract:
The Internet has provided IS researchers with the opportunity to conduct studies with extremely large samples, frequently well over 10,000 observations. There are many advantages to large samples, but researchers using statistical inference must be aware of the p-value problem associated with them. In very large samples, p-values go quickly to zero, and solely relying on p-values can lead the researcher to claim support for results of no practical significance. In a survey of large sample IS research, we found that a significant number of papers rely on a low p-value and the sign of a regression coefficient alone to support their hypotheses. This research commentary recommends a series of actions the researcher can take to mitigate the p-value problem in large samples and illustrates them with an example of over 300,000 camera sales on eBay. We believe that addressing the p-value problem will increase the credibility of large sample IS research as well as provide more insights for readers.
-------
Note: We published a working paper already in 2009, but have toned it a bit down in the review process... The working paper is available on SSRN.
"Directionally-Sensitive Multivariate Control Charts in Practice: Application to Biosurveillance" accepted for publication
Our paper, "Directionally-Sensitive Multivariate Control Charts in Practice: Application to Biosurveillance, co-authored with Inbal Yahav, has been accepted to Quality and Reliability Engineering International.