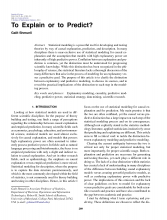
My research examines the fundamental and practical differences between using statistical and other empirical methods for prediction compared to causal explanation and to description. Although the discussion of explanation vs. prediction has been actively pursued in the philosophy of science, the statistics literature has not considered it in a holistic way. Yet, statistical modeling can be and is used for each of these goals. My thesis is that statistical modeling, from the early stages of study design and data collection to data usage and reporting, takes a different path and leads to different results, depending on whether the goal is predictive or explanatory.
The current conflation of explanation and prediction (in empirical modeling) is pervasive in many fields, and especially in the social sciences. Not only are explanatory power and predictive power confused, but there is a clear lack of adequate predictive modeling in those fields (also due to the under-appreciation of the scientific role of prediction).
My work is aimed at
- Explaining and formalizing the many aspects of empirical modeling that differentiate explanation and prediction,
- Clarifying the concepts of "explanatory modeling" and "predictive modeling", which mean different things to statisticians, social scientists, computer scientists and other scientists,
- Discussing the crucial scientific role of prediction (assessing predictive power and building predictive models), and
- Equipping "explanatory" scientists with a better understanding of how to carry out predictive modeling.
More information is available below.